Dear visitor!
Data are at the heart of our society, technology, and organizations. In fact without data, tools to collect and methods to analyze and interpret them our ancestors would not have been able to follow the traces of wild animals for food and to grow plants and breed animals later.
And in the course of this what later was named the First Agricultural Revolution (Neolithic Revolution) cities were build which led to further use of numbers: With taxation as one of the most important (and still is from the state points of view).
Tip: I have collected some important terms around data in German with links, so you might check this page out as well.
The Scientific Revolution
The progress continued slowly but surely and exploded at the end of the Middle Ages and the beginning of the Renaissance with data-based inventions and explorations by people like Nicolaus Copernikus (see this ARTE Documentary about him and his fellow Georg Joachim Rheticus), Johannes Kepler, Tycho Brahe, Galileo Galilei, as well as Isaac Newton and Gottfried Wilhelm Leibniz.
Exact and systematic observation of natural events, the collection of these data over a long period, thinking about these and developing theories, testing these with experiments as well as the exchange and correspondence with other researchers marked the start of the Scientific Revolution and its scientific method which paved the way to the Industrial and Digital Revolution on wich all our knowledge and wealth is based on until today.
“Standing on the shoulders of giants”, as Google often quotes, is as true as the onegoing efforts of many people today to solve the micracles of the universe and to answer open questions regarding our earth, our economy, and technological challenges.
Bricks without clay
“Data! data! data!” he shouted impatiently. “I can’t make bricks without clay.”
These words come from the mouth of Sherlock Holmes and the short story “The Adventure of the Copper Bleeches” by Arthur Conan Doyle, which was first published in The Strand Magazine in June 1892 – and which also appeared in the anthology “The Adventures of Sherlock Holmes” in October of the same year.
But let’s the master detective from London himself speak:
Although the famous detective from London is a fictional character, for his time and even today, his approach is a prime example of how to solve a difficult task, a puzzle and a case: through precise observation, the collection of data, scientific methods and logical reasoning (deduction). In other words, a forerunner of the data scientist!
And every data scientist or people analyst – like Sherlock Holmes – needs data! Data! Data! Fortunately, technological progress since the 1990s with hardware such as computers, chips, the internet, smartphones and increasingly powerful software has led to huge mountains of data from which the valuable “data ore” now needs to be mined (technically: data mining).
Data as ores for knowledge and wisdom
Even if data does not have quite the same material significance as oil or gold, a comparison we read more often, it is still central to making decisions and translating results into action when it comes to the right selection, cleansing of raw data (interesting: similar to ore as a metal or mineral mixture and raw material), analysis and visualization.
Tip 1: See also the data science pyramid (DIKW) with the levels from bottom to top: World → Data → Information → Knowledge → Wisdom; (see e.g. Herter, 2022, “Was ist Data Science?”, p. 25, in Wawrzyniak & Herter (Eds.), Neue Dimensionen in Data Science: Interdisziplinäre Ansätze und Anwendungen aus Wissenschaft und Wirtschaft, Berlin – Offenbach: Wichmann/VDE). Note: Michael Herter is CEO of Bonn based data science company infas 360 GmbH.
Tip 2: A short practical book on data science in German has written Michael Oettinger (2020).
And: For Germany you might take also a look on the work of the German Data Science Society (GDS e. V.) and their event German Data Science Days taking place from March 7-8, 2024 in Munich. See also upcoming events of the Data Science Summit.
Origin of data I: Classification
Anyone who practices data science or people analytics (HR data science) therefore needs data. But where exactly does it come from? What sources are there? And how available is it?
Of course, organizations today primarily generate mass data (big data) as well as smaller amounts of data (small data): Here, data can be differentiated according to who or what it basically comes from and where it originates, such as:
- Data from nature and agriculture (e.g. weather, soil, animals, plants)
- Data from technical systems and machines (e.g. power plants, factories, vehicles)
- Data from the economy, corporate management and the financial sector (macroeconomic figures, key business figures, taxes).
And what I am interested in as an HR data scientist or people analyst: data from people. More precisely: data from people in organizations – i.e. from employees, managers and trainees (HR data).
There are a number of other ways of classifying data, such as raw data, aggregated data or metadata, according to data type or file format or authorizations. However, it is important that such data classification takes place in accordance with the existing guidelines and is checked over time.
This is always personal data under data protection law, as is the case with customer data or patient data, which is subject to special legal protection.
Personal data also includes data that can be used to identify a person with reasonable effort, such as the license plate number, the account number or the personnel number, which are often used in databases as so-called primary and foreign keys.
Origin of data II: Internal sources
But let’s leave these information technology and legal aspects behind and return to the initial question: Where does the data come from?
Because as I said: (HR) data science and people analytics need to develop and implement solutions to HR challenges: Data.
Fortunately, a large amount of data is collected and stored within an organization today, which, together with other internal or external data, is available to the employee or external service provider for analysis.
Well, in the case of data science or people analytics projects, we usually have access to this data – even if it often involves a lot of effort, communication and processing; as well as to the relevant data sources of interest from business and human resources management such as databases, data warehouses or data lakes (or other modern architectures such as data lakehouses or data meshes). The relevant data is often also available as files (flat files) in various formats (e.g. cvs, xls, xml, json).
The development of data systems, the storage and use of data (transformation, extraction) is summarized under the term data engineering, which has led to the profession of data engineer, as the complexity of IT systems and the challenges posed by big data, IT system landscapes, software diversity and cyber security, for example, have grown significantly in the last 10 years.
The data from Human Resource Management (HR data for short) includes, for example, personnel master data and applicant data, wage and salary data, data on sick leave and fluctuation, data on qualifications and further training (e.g. e-learning) or on job satisfaction and employee management.
In the case of internal data from other areas of the company, HR data science and people analytics projects may be interested in communication data, company figures or working hours (e.g. overtime), depending on the issue at hand.
However, there are situations in which we do not have access to company data, but still need it for testing, training or demonstration purposes. What can we do? The solution: Public or open data!
Tip: For a comprehensive overview of these internal data sources and data from third parties (external data), see the short and practical reference book by Steffi Rudel (2021).
Origin of data III: External sources
There are a lot of external sources for data available which allow access of public or open data.
However, while there are many Internet offerings for a lot of data from politics, society, the environment, transport and health, to name but a few, real data on human resource management is very rare for obvious reasons of data protection and company secrecy.
However, there are some real and fictitious HR data sets that can be used for various purposes for data science and data analysis. For example, for practicing and learning, for testing hypotheses or for comparison with your own HR data.
External data from public, general and special sources with data on the labor market, employer ratings, customer satisfaction, demographic characteristics or the industry and market are also used for specific questions in an HR data science or people analytics project.
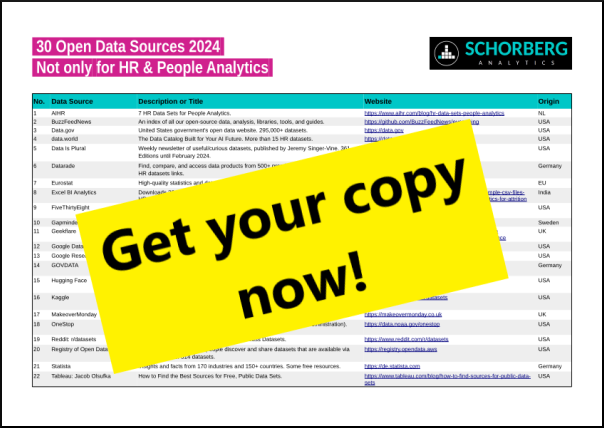
Schorberg Analytics and Stefan Klemens have collected 30 sources of public and open data in a PDF, which also contains links to a number of HR datasets: If you are interested in this collection contact Stefan Klemens via contact form, e-mail or LinkedIn message. [Please connect there and like three of my latest post, if you have not yet, or comment on it. Friends and supporters of Schorberg Analytics and Stefan Klemens get the PDF of course immediately!].